
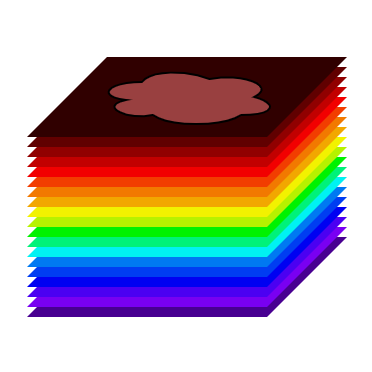
While researching deeper into the use of robotics in agriculture, a topic of interest to me, I came across spectral imaging. This page serves as an organized compilation of my findings on spectral imaging, with a specific focus on its applications in agriculture. Part of this research was conducted for a competition project - an autonomous weed and disease identification and mitigation/elimination rover - for the AMD Pervasive AI challenge hosted by AMD and Hackster.
Spectral imaging is imaging over multiple bands in the electromagnetical spectrum, combining elements of spectroscopy with traditional photography. In spectral imaging, each pixel in the captured image contains the intensity information from various bands of the electromagnetical spectrum, offering more insights into the object of study, wether it is the Earth’s surface viewed from space or individual plants in precision agriculture. Different spectral imaging technologies, both active and passive, along with various platforms like satellites, drones, and rovers, are employed depending on the application.
Technically an ordinary camera can be considered a spectral imaging system, as it captures images in three spectrums (for the three colors: red, green and blue), the term “spectral imaging” is typically reserved for scenarios where more then just those RGB bands are acquired. Additionally, the RGB filter used in a ordinary camera, known as the Bayer filter, is not narrowband, allowing the passage of light of neighboring bands. It is after all tuned to closely mimic the spectral response of the human eye. Also, multispectral imaging often extends beyond the visible region, both into higher wavelengths such as Near-Infrared (NIR) and Far-Infrared (FIR) for thermal imaging, as well as lower wavelengths including Ultraviolet and even X-Rays.
Spectral imaging can be classified into two categories: Multispectral or Hyperspectral imaging, depending on the number of acquired bands. While there is no exact cutoff between the two, a general guideline is to consider an imaging system multispectral if it acquires fewer than 15 bands and hyperspectral if it acquires more. Typically, a “package” or set of images, on for each spectral band, is organized into a data cube, a multi-dimensional array of values in a 3D format representing N bands of 2D images, illustrated in the figure below.
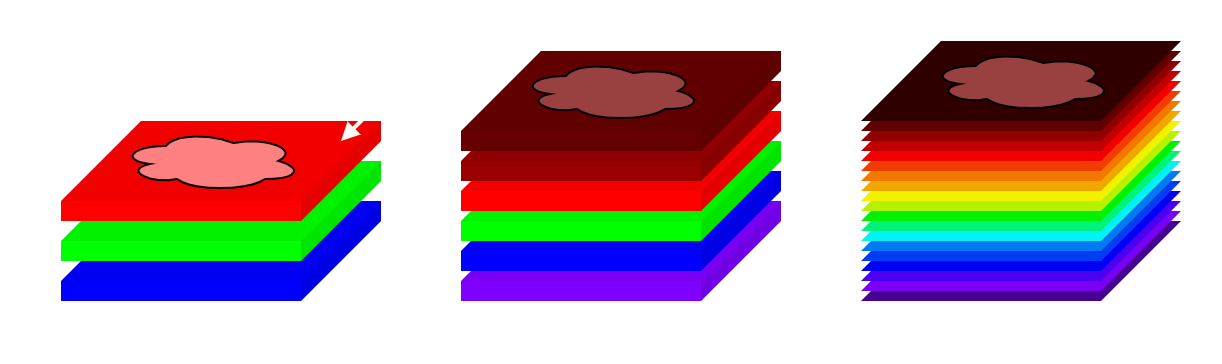
Looking at the above data cube in the vertical direction, each slice or band represents the spectral response for every pixel in the 2D image. The exact spectrum captured in each slice or band varies depending on the imaging system utilized. Below is a figure representing an example spectral response for both multispectral and hyperspectral systems, alongside a typical spectral response of an RGB camera using a Bayer filter.
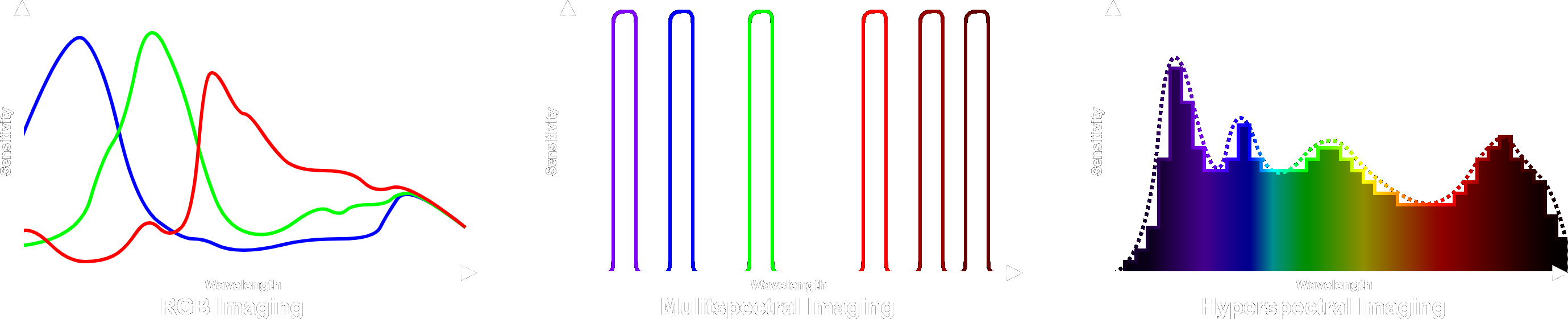
This comparison highlights the difference between multi- and hyperspectral imaging, particularly in terms of the number of acquired bands. In a hyperspectral system, the acquired bands can span an almost continuous spectrum. It also shows the big difference of the narrow and sharply defined cutoffs with little to no overlap bands of spectral imaging, in contrast to the wideband and non-uniform characteristics of the RGB camera’s spectral response.
A spectral imaging system can be characterized, under others, by the following parameters:
- Spatial Resolution: Either the number of pixels, the resolution, of a single image, or the square area each pixel represents in the real world surface.
- Spectral Resolution: The number of different bands that can be distinguished.
- Radiometric Accuracy: How accurate the system is e.g. how accurate the spectral reflectance can be measured.
Spectral imaging is used in a wide array of applications across various domains, spanning from laboratory settings in biology and material science to earth observation for biome classification and resource identification, and agriculture for pest detection and crop health assessment. Focusing on the topic of interests to me, the two main areas are: earth observation, particularly in terrain characterization and biome identification, and agriculture, where spectral imaging plays a crucial role in pest and disease identification, crop classification, and yield estimation.
Earth Observation
Starting with the more general area, application of spectral imaging in earth observations. Here, spectral imaging serves as a powerful tool for identifying and classifying large outdoor areas, like terrains, urban landscapes, and industrial zones. Typically conducted with satellites, high-altitude planes, or balloons, these applications operate with lower spatial resolutions, with pixel sizes in the range of square meter or larger. Earth observation applications can broadly be categorized into two areas: geology and surveying, and environmental monitoring.
In geology and surveying, spectral imaging aids in terrain classification, soil mapping, biomass/vegetation assessment, and identification of the species in the vegetation. This is achieved by analyzing the reflectance characteristics of different terrains and vegetation types. Below is a simplified example showing the reflective spectral response of various land surfaces, as well as the differentiation between healthy and unhealthy vegetation.
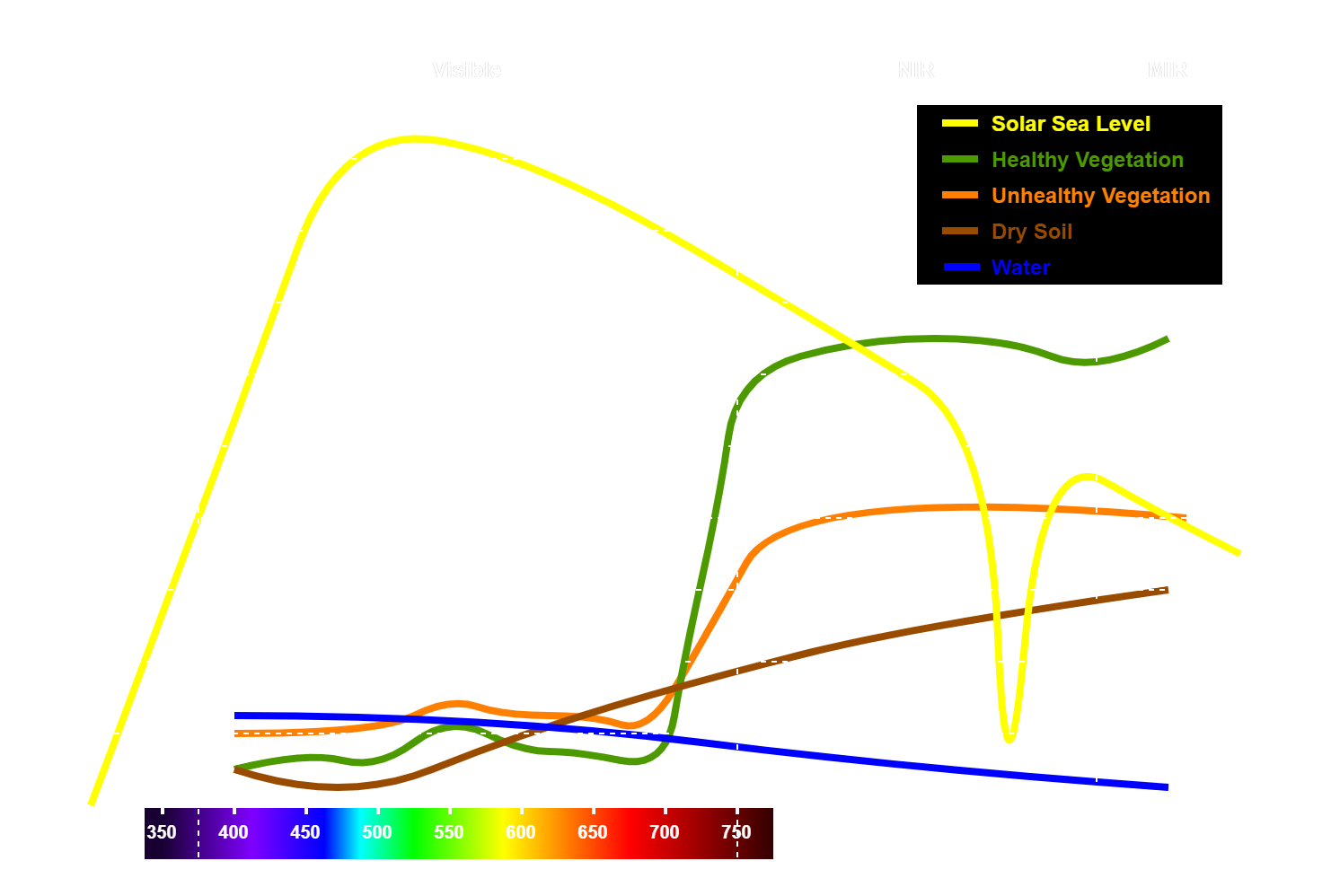
From this, and from a more complete analysis of reflectance characteristic, the major spectral bands of interest in this application can be identified:
- 450nm: For costal areas and marine environments (Unaffected by atmospheric scattering)
- 500nm: For distinction of soil from vegetation and distinction of deciduous from coniferous (Unaffected by atmospheric scattering)
- 550nm: The reflectance peak for healthy vegetation, also helps in plant classification
- 600nm: For plant and soil classification, detection of materials in soils and identifying yellowing of foliage
- 650nm: Main band of absorption by chlorophyll, detection of soil and geological features
- 720nm: Important in identifying plant health as the reflectance of this bands in vegetation depends strongly depending on chlorophyll content
- 800nm: For identifying moisture content of biomass
- 900nm: Emphasizes biomass content and shorelines (Weak influence from atmosphere)
In environmental monitoring, spectral imaging is used in detecting and tracking various pollutants and hazardous substances in water and soil, as well as identifying and classifying hazardous waste. This includes monitoring activities such as detecting and tracking pollutant spills, and assessing environmental impacts. It also aids in monitoring landfills and identifying and tracking wildfires. This is a very important area, specially these days where we are faced with an environmental crisis so helping identify disasters faster and more accurately is essential.
Agriculture (Precision farming)
In agriculture, the use of spectral imaging offers detailed insights into crop fields with a level of granularity that can extend down to individual plants. There is quite some overlap with earth observation applications, such as terrain identification, soil mapping, and biomass assessment, but agricultural applications require higher spatial resolution and more frequent scans/updates. Therefore, the surveys are typically conducted using low-altitude planes, drones, and ground-based vehicles like rovers or tractor pulled riggs.
Also here, it is important to analyses the reflectance properties of different materials and crops as well as the change of it with healthy vs unhealthy crops. Commonly used spectral processing techniques in agriculture applications include vegetation index (VI) calculation, such as the Normalized Difference Vegetation Index (NDVI). NDVI calculates the ratio of the difference between near-infrared (NIR) and red bands, often in the red edge band. This because here the sharpest change of vegetation reflectance occurs, due to absorption by chlorophyll (for photosynthesis), as was shown in a figure of the previous section. To highlight this effect in more detail, the figure below illustrates the absorption spectrum of different types of chlorophyll.
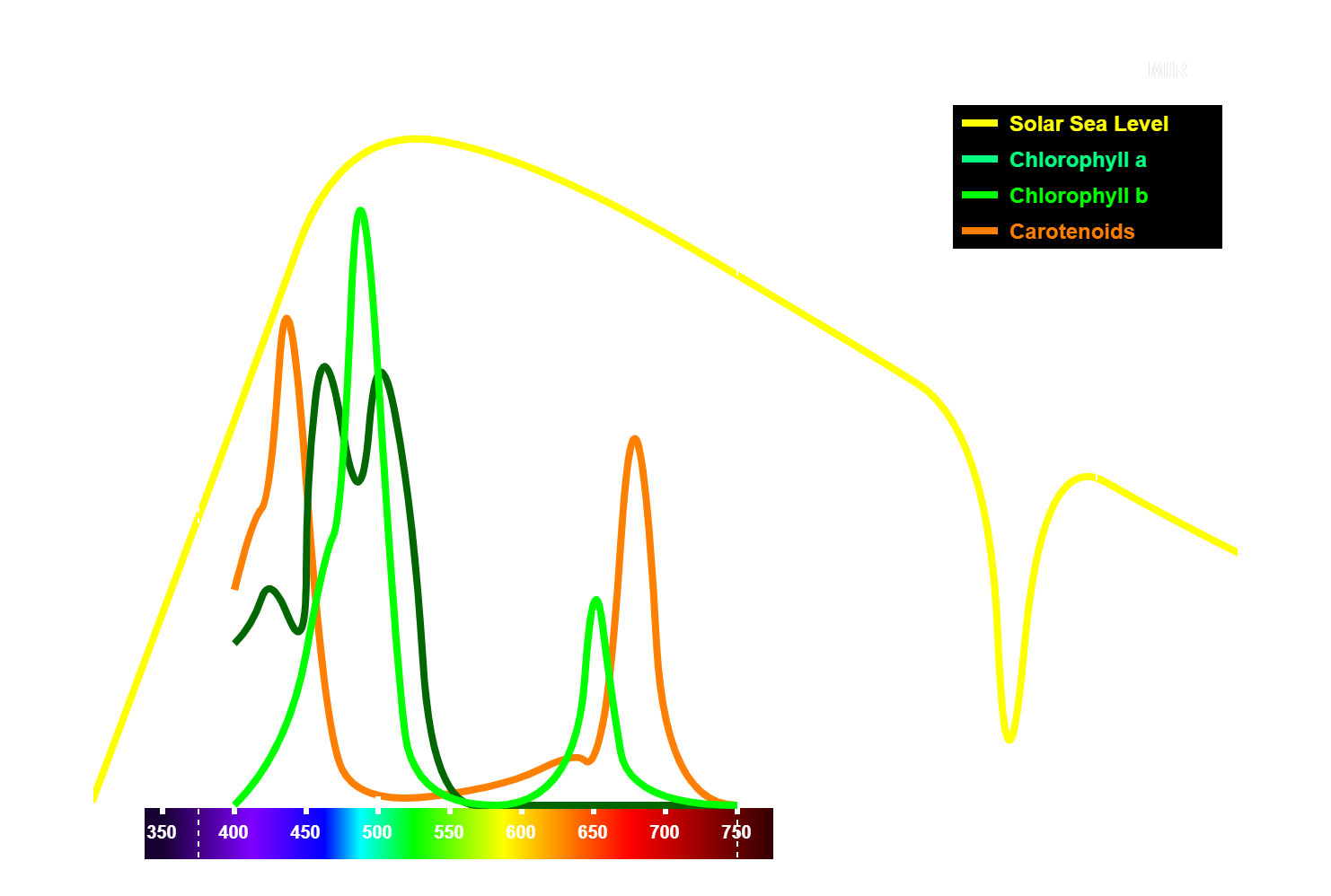
Spectral images collected using these techniques enable a diverse range of assessments in agriculture, including:
- Determining fertilization by identifying symptoms of nutritional deficiencies
- Optimizing pesticide application through early detection of biotic stress
- Fine-tuning irrigation by identifying areas of potential water stress
- Calculating agronomic indices and prepare monitoring plans to forecast crop yields
These applications are essential in agriculture, enabling increased yields while reducing pesticide, fertilizer and water usage, thereby optimizing costs and minimizing waste and environmental impact. This enables precision farming, a steadily growing area, which harnesses the power of spectral imaging alongside other environmental sensors, edge-processing technologies, and machine learning models to formulate optimized action plans based on comprehensive sensor data. This data-driven farming approach improves upon traditional agricultural practices, enabling farmers to make better informed decisions,enhancing productivity and sustainability at the same time.
Others
Besides the two applications of spectral imaging mentioned above, it finds use in a variety of other fields and industries. Here is a short list of a several additional applications:
- Astronomy: Analyzing the emitted or reflected light from celestial bodies across different wavelengths, enabling insights into their composition, temperature, and movement.
- Biomedical imaging: Provides detailed information about tissues and cells, aiding in disease detection, monitoring, and treatment assessment.
- Chemical imaging: Allows the identification and visualization of chemical compounds and their distribution in various samples, facilitating applications in pharmaceuticals, forensics, and environmental monitoring.
- Civil engineering: Aids in assessment and monitoring of infrastructure, enabling the detection of structural defects, material degradation, and environmental impacts on buildings and bridges.
- Food processing: Helps ensuring food quality and safety by detecting contaminants, assessing ripeness, and monitoring processing stages such as cooking and drying. Is related to agricultural applications.
- Surveillance: Specially NIR and far infrared for nightvision and thermal vision applications.
- Waste sorting and recycling: Facilitates automated waste sorting by identifying and categorizing different materials based on their spectral signatures, improving recycling efficiency and reducing landfill waste.
Spectral imaging can be achieved through a variety of methods, each with its own level of complexity and applicability. The most prevalent in commercial multispectral imaging solutions uses specialized bandpass optical filters, a filter-based multispectral imaging. The implementation of multiple filters can vary, ranging from filter wheels to independent cameras with a single spectral band filter. Another technique is using active illumination instead of optical filtering. There are also less common and more complex systems using tunable filters and spectral scanners, mostly used in Hyperspectral imaging systems, but they lay outside the scope of this page (maybe worth revisiting at a later time…).
Active Illumination
Active multispectral imaging systems utilize sources of illumination, such as LEDs or lasers, emitting light at specific wavelengths of interest. By controlling the illumination source, these systems selectively illuminate the scene with different bands of light and capture the reflected image with typically a Mono (BW) CMOS sensor, without the need for any optical filter. Because spectral band selection relies solely on the emitted light from the light source, no optical filtering on the capture side, this type of imaging requires minimal to no ambient light, which could otherwise be reflected by the object and captured by the imaging system. This means that this type of spectral imaging only works in a controlled lighting environment and for close-range objects, it is unsuitable for aerial or satellite based imaging. However, because the lighting conditions are by design well controlled, it does not require special spectral sensors for compensating for environmental lighting condition changes, as their should be none. Also, this system is cheaper as narrowband illumination, specially using LEDs, is less expensive than narrowband optical filters. It is therefore a good option for imaging in the laboratory or outdoors at night on a rover platform.
An example of a multispectral illumination system, used for active spectral imaging, is the system manufactured by Spectral DEVICES and which is shown in the image below.
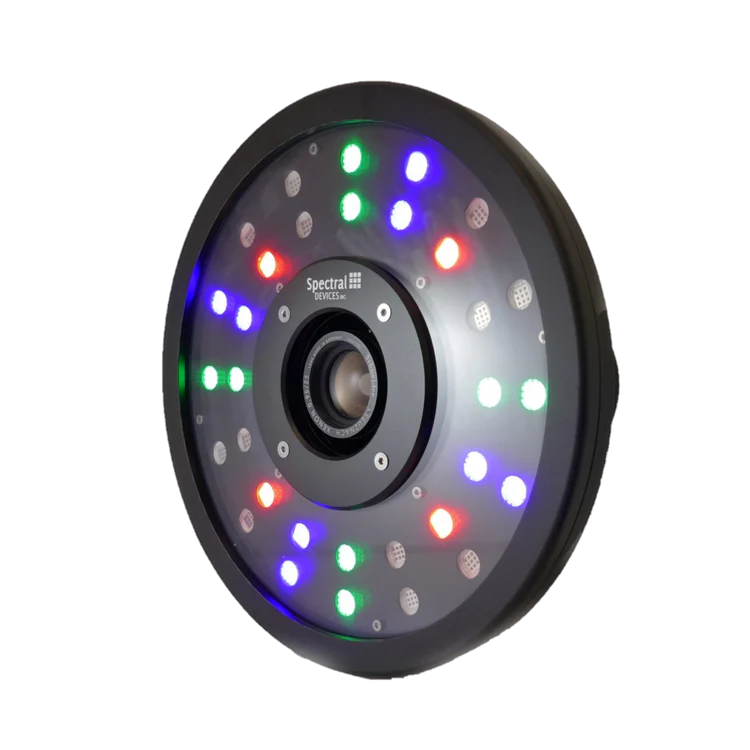
Filter Wheel
In discrete optical setups, such as optical test benches and similar, as well as in space fairing imaging, the spectral selection is performed with a filter wheel. This filter wheel houses an array of optical filters, each designed to isolate or exclude specific optical bands of interest (or to attenuate light with OD filters). Imaging is accomplished using a single high-performance Mono (BW) CMOS sensor, offering efficiency and simplicity in data acquisition. Similar to the active illumination method, a single high-performance camera and lense is required but unlike that method, here the band selection is achieved through optical filtering instead of the selective illumination. Optical filter wheels are typically larger in size, making them suitable for use in large imaging systems such as satellites or ground-based optical telescopes. The image below illustrates a compact filter wheel designed for telescope-based space imaging.
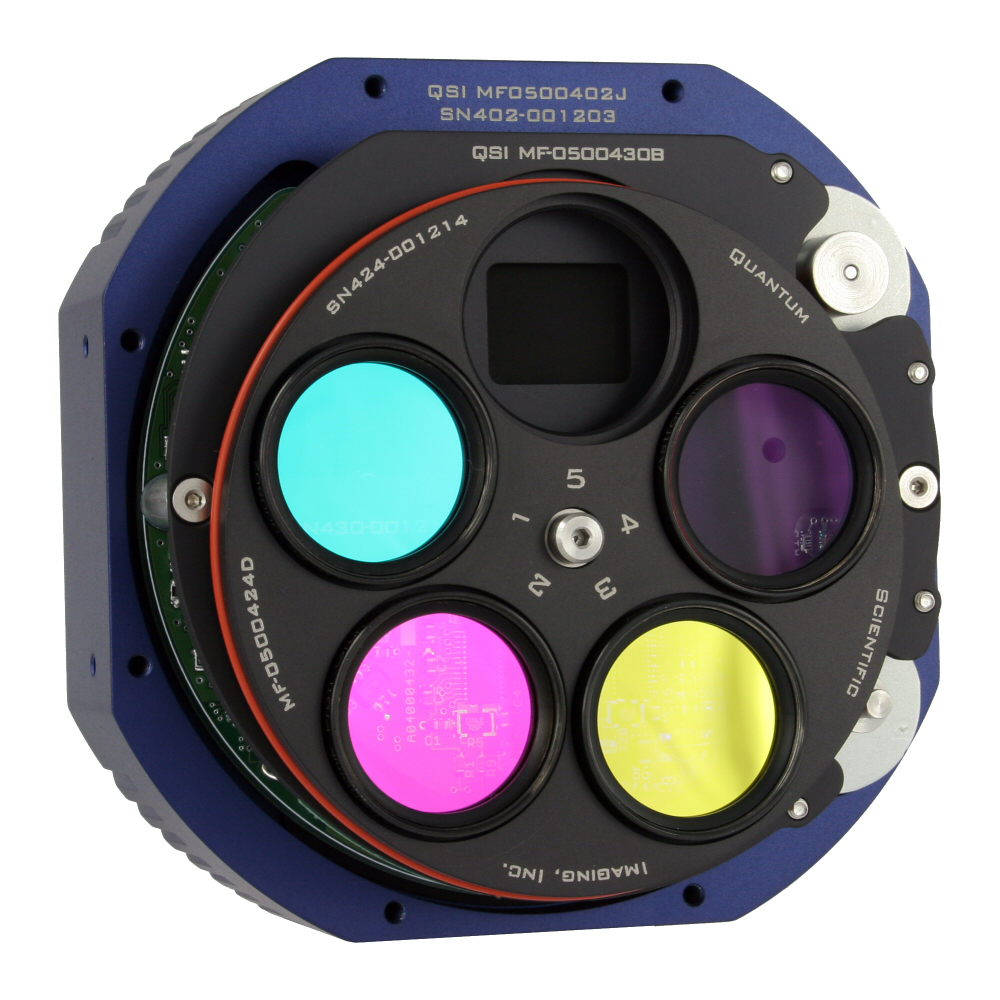
As already mentioned, this type of imaging is mostly used in space fairing imaging (e.g. in the Hubble Space Telescope and the James Webb Space Telescope) and ground telescopes, where specialized high-performance large optical sensors are employed and therefore the use of a single sensor is preferred. Additionally, the use of a single sensor ensures consistency in image capture across all spectral bands. Notably, there are no spatial differences in the acquired images from different bands, as they are all captured by the same sensor and through the same lens.
Multi-Camera
One of the most common methods used for constructing a multispectral imaging system involves employing multiple image sensors, each equipped with its own narrowband optical filter. This configuration offers a mechanically simple system, eliminating the need for filter changes, and enabling (time) synchronous acquisition of all bands. To get the best performances, these systems typically use global shutter Mono (BW) CMOS sensor, ensuring a rolling-effect-free and synchronized acquisition of all sensors (bands) simultaneously. Below is a table comparing several commercial multi-camera multispectral imaging solutions (as of May 2024).
Company | Model | Sensors | Size/Type | Resolution | Bands (nm) | FoV | FPS | Price ($) |
---|---|---|---|---|---|---|---|---|
DJI | P4 Multispectral | 1 RGB + 5 MS | 1/2.9" Global | 2.08M | B (450), G (560), R (650), RE (730), NIR (840) | 62.7D | ? | 6'499 |
AgEagle | MicaSense RedEdge-P | 1 RGB + 5 MS | Global | 5.1M + 1.6M | B (475), G (560), R (668), RE (717), NIR (842) | 44H 38V | 3 | 7'995 |
AgEagle | MicaSense Altum-PT | 1 RGB + 5 MS + 1 TH | Global | 12M + 3.2M + 320x256 | B (475), G (560), R (668), RE (717), NIR (842) | 50H 38V | 2 | 15'995 |
Yusense | AQ600 | 1 RGB + 5 MS | 1/1.8" Global | 12.3M + 3.2M | B (450), G (555), R (660), RE (720), NIR (840) | 48.8H 37.5V | 1 | 12'000 |
Yusense | MS600 | 6 MS | 1/3" Global | 1.2M | B (490), G (610), R (650), RE (780), NIR (940), Other | 49.5H 38.1V | 1 | 7'400 |
Parrot | SEQUOIA | 1 MS + 4 MS | Global | 16M + 1.2M | G (550), R (660), RE (735), NIR (790) | 61.9H 48.5V | 1 | 3'499 |
Sentera | 6X Multispectral | 1 RGB + 5 MS | Global | 20M + 3.2M | B (475), G (550), R (670), RE (715), NIR (840) | 47H | 5 | 12'000 |
MAPIR | Kernal2 | 6 MS (Modules) | 1/1.8" Global | 3.2M | Configurable (26) from 342nm to 915nm | 87H or 41H | ? | 15'500 |
MAIA | MAIA WV (or S2) | 1 RGB + 8 MS | Global | 1.2M + 1.2M | V (423), B (488), G (550), O (603), RE (725), NIR (785), NIR (888) | 35H 26V | 6 | 14'500 |
MAIA | MAIA M2 | 2 MS | Global | 1.2M | Configurable (17) from 423nm to 888nm | 36H 27V | 1 | 2'500 |
As can be seen from this table, most commercial systems can capture 5 different spectral bands using 5 sensors, alongside one RGB sensor, often with a higher resolution. This setup results in a high cost, as it requires 5 nearly identical optical systems and image sensor differing only in the filter used. However, they offer mechanical robustness with no moving parts, and they are quite compact. Compared to an active illumination setup, they excel in aerial-based imaging applications. Below is a gallery of pictures of the imaging systems listed in the table above.
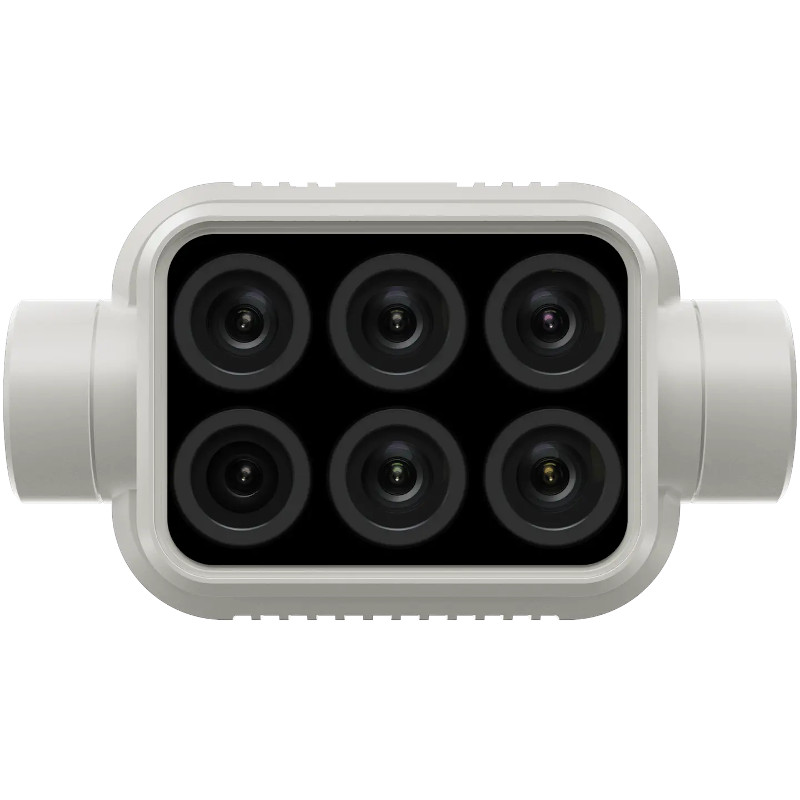
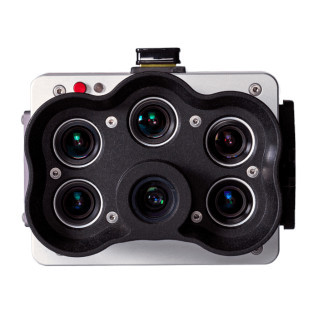
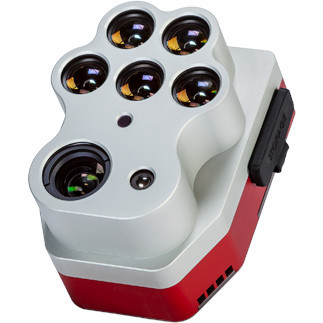
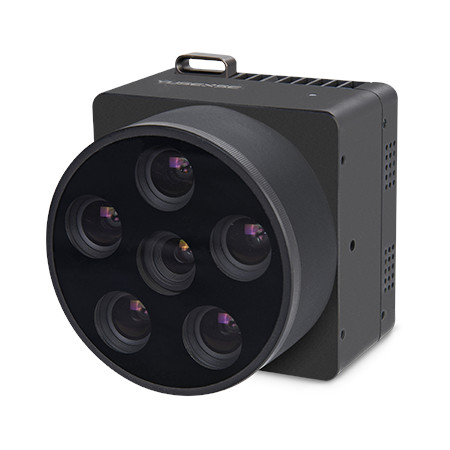
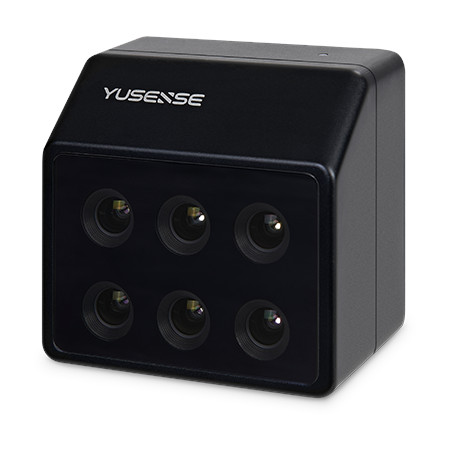
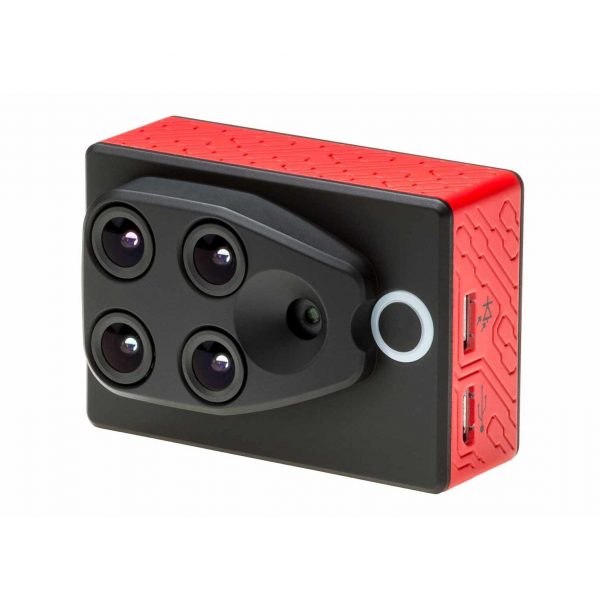
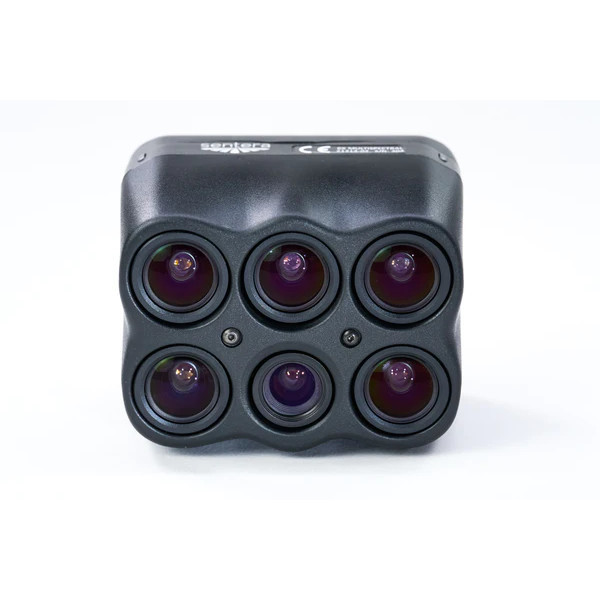
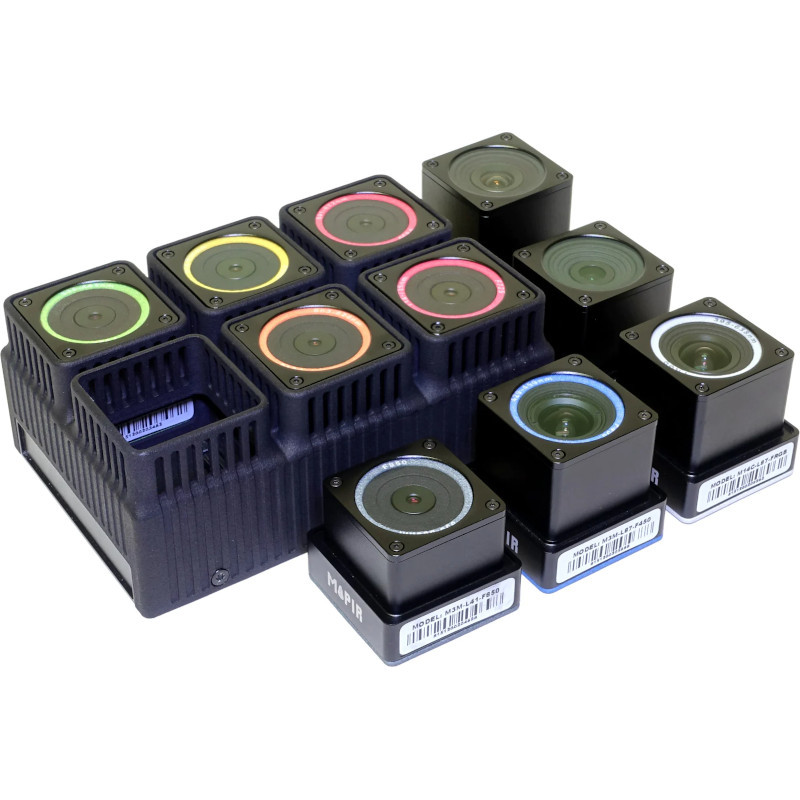
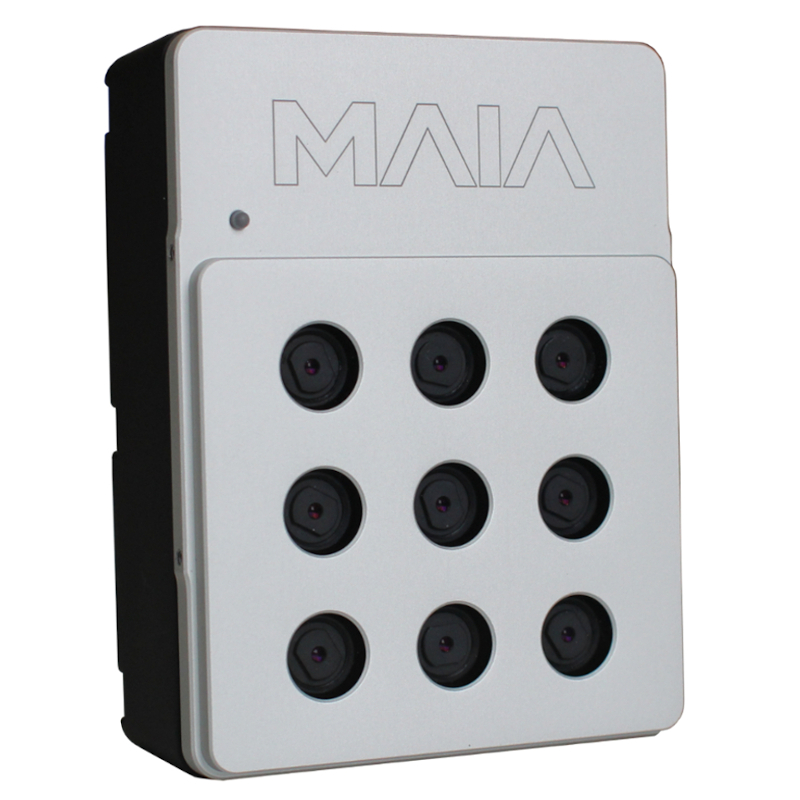
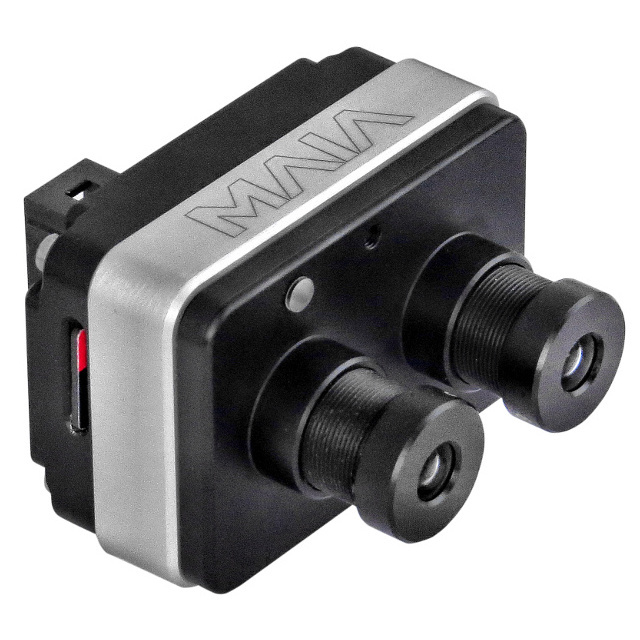
Multi-Band Filter
An interesting alternative to a multi-camera setup is to use a multi-band filter in combination with a RGB CMOS sensor. In this approach, the added multi-band filter selects up to three spectral bands of interest, while the RGB camera’s integrated Bayer filter separates these bands into individual channels (R, G and B). This reduces the number of required sensors by a factor of 3, offering a more compact and cost-effective solution. However, it comes with a compromise in spectral purity, as the RGB camera’s Bayer filter are not very narrow, allowing light of neighboring color bands to pass. This is especially true when the selected bands are close together or fall in a region where the Bayer filter permits light to pass through for all color pixels, such as in NIR region. This type of multispectral imaging is used by MAPIR with its single single sensor Bayer-RGB solution, the Survey3.
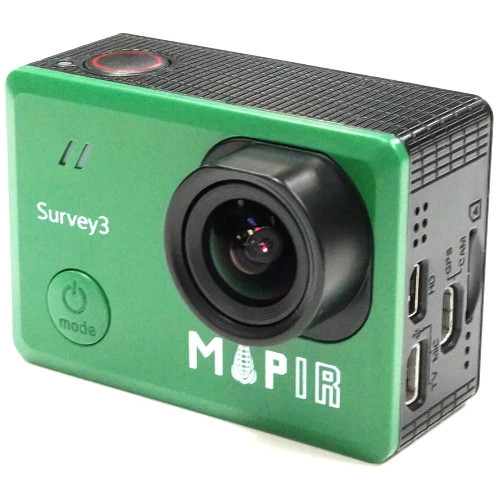
The big advantage of this solution is its cost-effectiveness, a 3 band imaging system can be bought from MAPIR for around 500 $.
Multi-Filter Lense
Another interesting approach is using a lense with multiple discrete optical filers. In this setup, a specialized lense contains multiple individual sub-lenses, each equipped with its own distinct optical filter corresponding to a specific spectral band. These sub-lenses focus their respective bands onto different areas of a large single CMOS sensor. Similar to the multi-band filter approach, this configuration enables the acquisition of multiple bands with a single Mono (B/W) CMOS image sensor. However, unlike the multi-band filter approach, this method avoids the issue of band leakage, as each band is well defined by its own discrete optical filter and does not rely on a Bayer filter in the image sensor. This type of multispectral imaging is implemented by Agrowing in its multispectral lense integrated into the Alpha 7CR Sextuple.
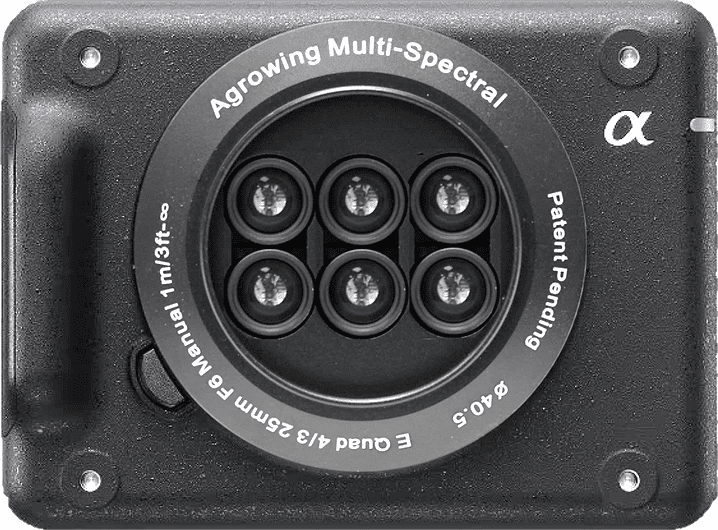
This specific solution employs a discrete and separate lense setup from the imaging system, allowing the use of a commercial high-performance camera, such as the Sony A7CR, as long as they have the same lense mount (in this case an E-Mount). This innovative approach combines the flexibility and image quality of professional-grade cameras with the spectral diversity enabled by multispectral imaging, offering a versatile solution for a range of applications.
Depending on the specific application, spectral imaging is performed with a wide variety of methods, as outlined in the previous section, and leverages various imaging platforms. In landsurvey, terrestrial imaging, and agriculture applications, remote imaging systems are the most common, either aerial (planes, drones, helicopters, etc…) and space-based. These offer fast imaging of large areas, but with limited spatial resolutions. When finer spatial resolution is required, ground based imaging platforms are employed e.g. to survey each crop specimens instead of a field wide overview.
Satellite
The use of satellites for landsurvey and terrestrial imaging is widespread as it provides a global coverage and once deployed can operate autonomously. It provides an ideal way of achieving long term imaging of large areas as the imaging satellite stays in orbit for multiple years, enabling the imaging of areas over long periods. They also offer a reasonably high update rate, most imaging satellites constellations visit the same area of land multiple times a month, some even daily. Both ESA and NASA provide a open to public satellite based multispectral imaging service, with the Sentinel-2A and 2B and Landsat satellites respectively. There are also some commercial offerings from MAXAR with the WorldView satellites and from Planet Labs with the SuperDoves constellation, providing (at a cost) higher resolution and faster update rates. Below is a comparison of these different satellite based multispectral imaging systems, showing the different available spectral bands in each system.
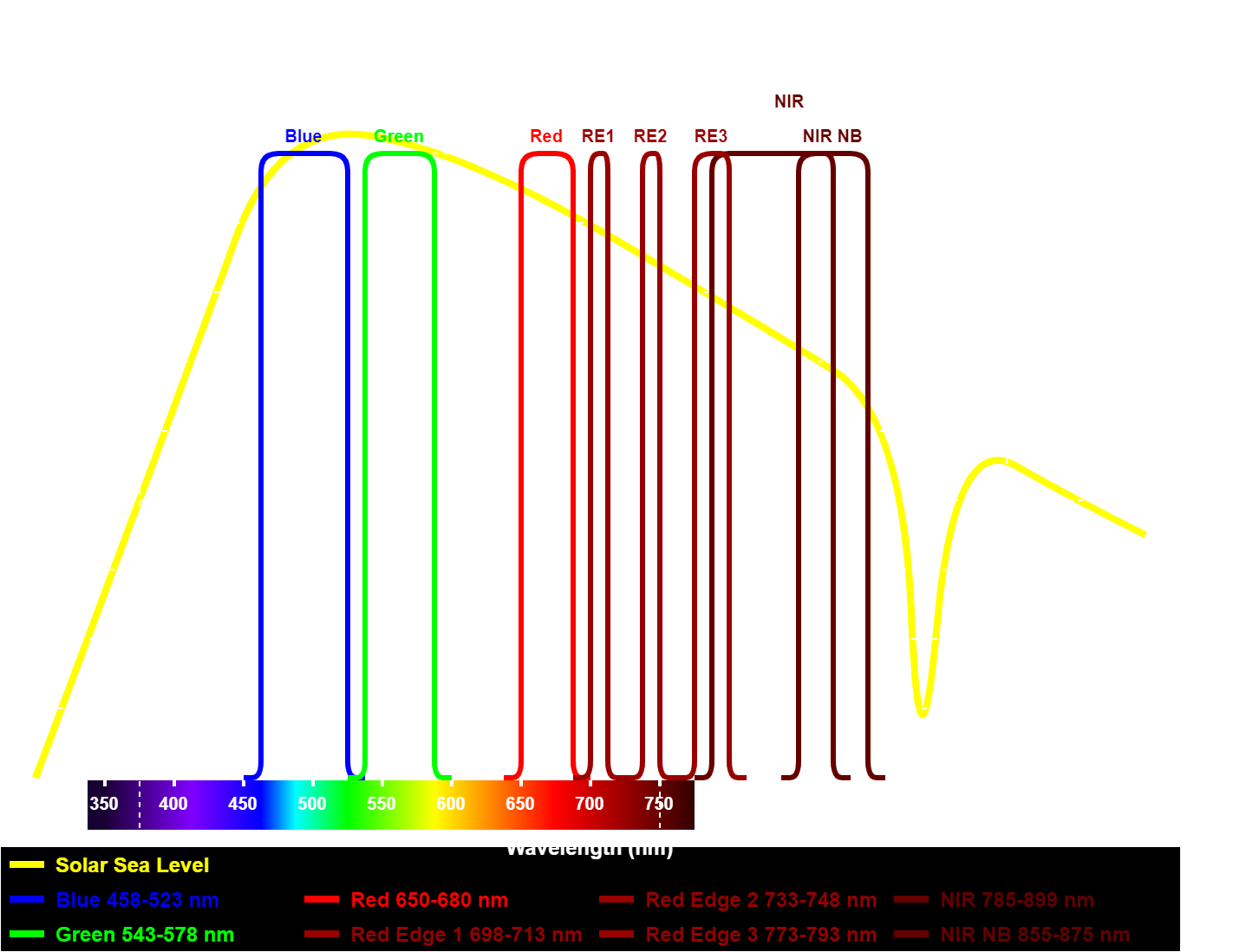
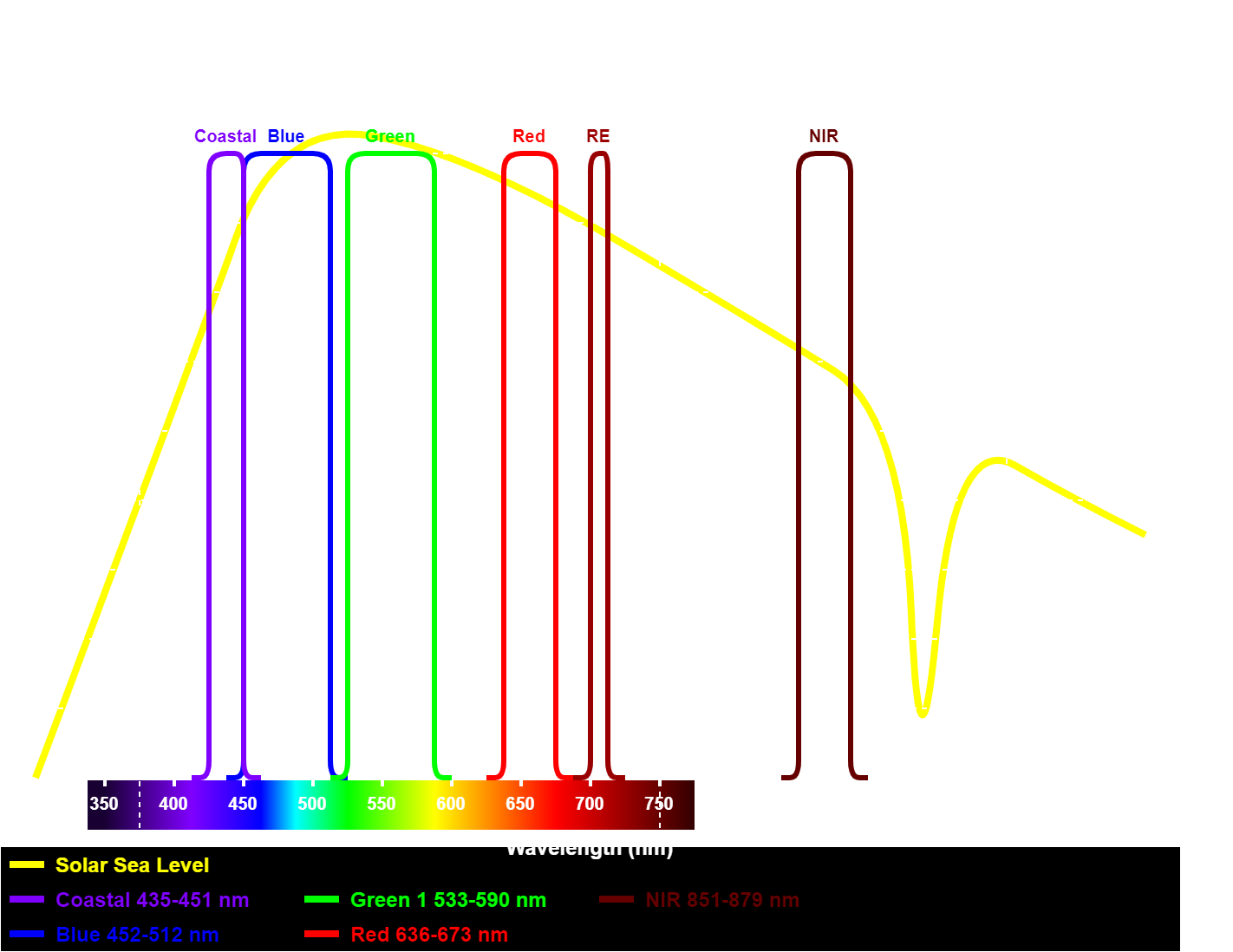
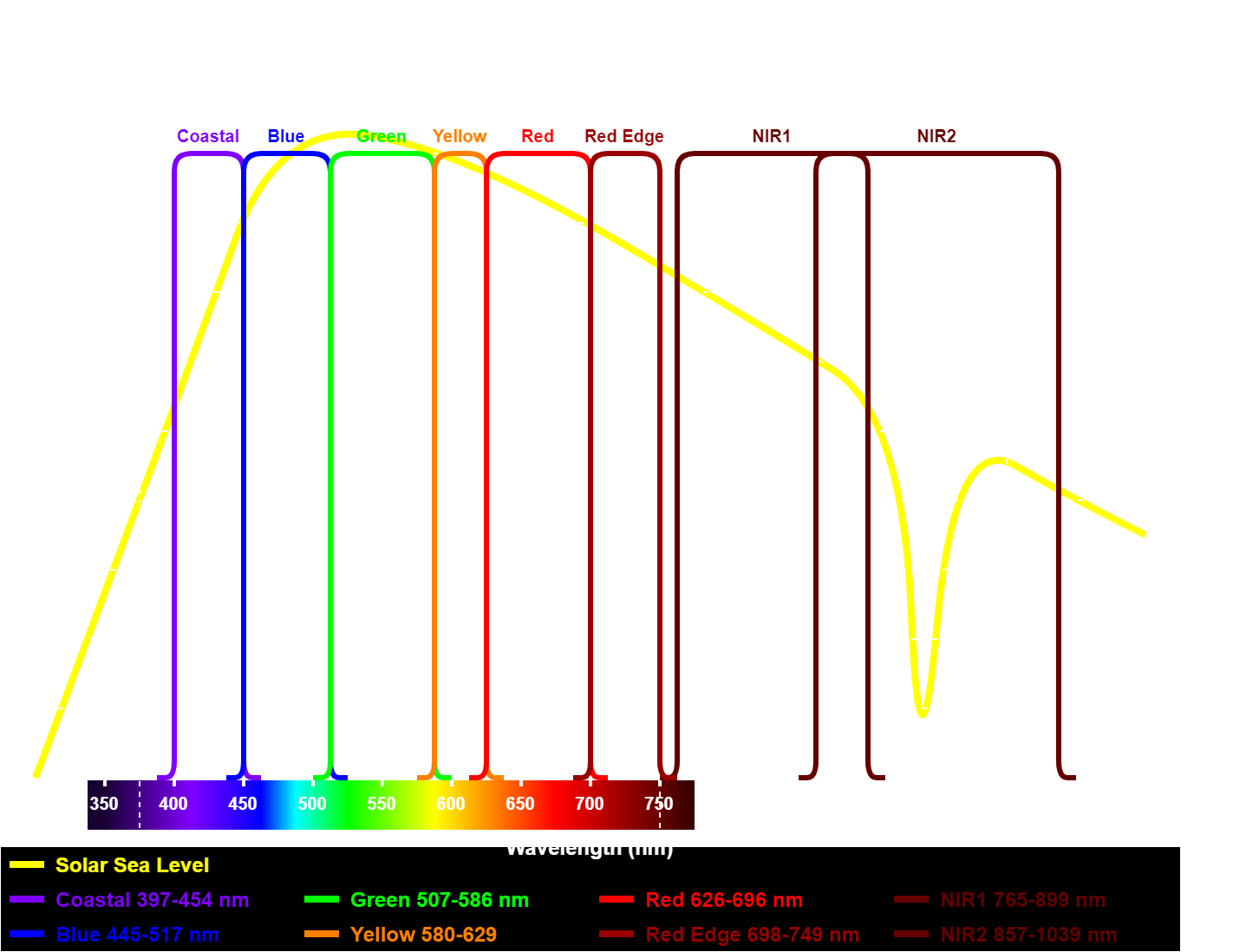
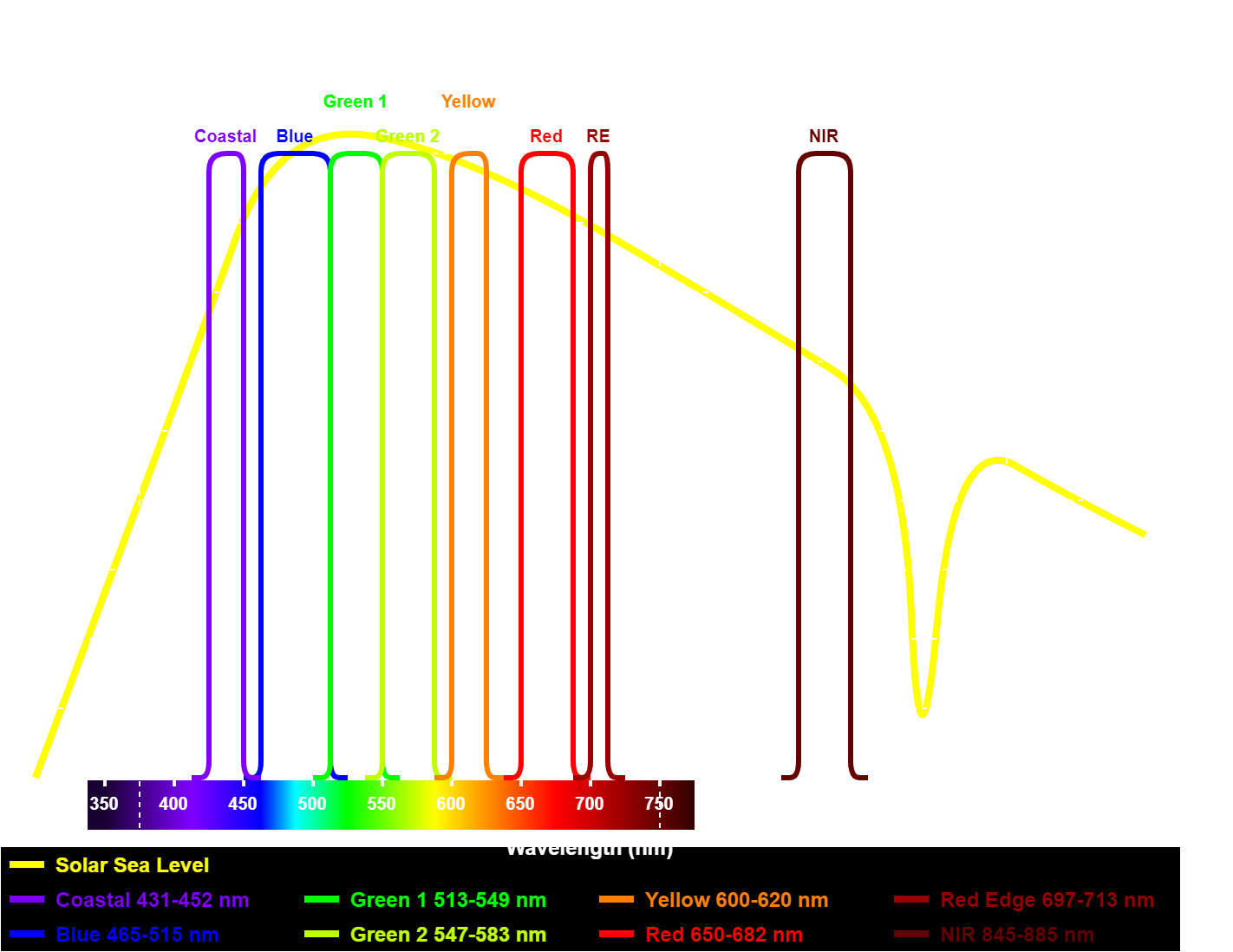
Aircraft
As seen above, satellites provide a great, and sometimes even free, multispectral imaging of large areas. However, their spatial, typically around 1x1 meter or higher, may not be good enough for many applications. For instance, in agriculture, while satellites provide a broad overview of crop field coverage across a country, they lack the detail required for farmers to effectively evaluate their fields. Additionally, space based imaging systems provide a fixed set of spectral bands, limiting flexibility in band selection and are heavily affected by atmospheric conditions like haze and cloud covering. This is where aircraft based imaging comes in, providing not only higher spatial resolution but also allowing custom imaging systems, e.g. custom bands, and flexibility of when and where to acquire the images.
Aircraft based systems use all types of aircraft’s such as planes, drones, helicopters and balloons. Planes are particularly useful for surveying large areas with high-precision systems, which are often large and heavy. However, they are large and expensive to operate, and drones emerged as a more cost-effective and versatile choice (as in MANY other applications, drones are amazing). Most drones can accommodate compact multispectral cameras, such as those listed in the previous section, as a payloads, forming relatively inexpensive and simple aerial multispectral imaging solutions. There are also off-the-shelf multispectral drones, like the DJI P4 Multispectral and the newer, more compact DJI Mavic 3M, depicted in the figure below.
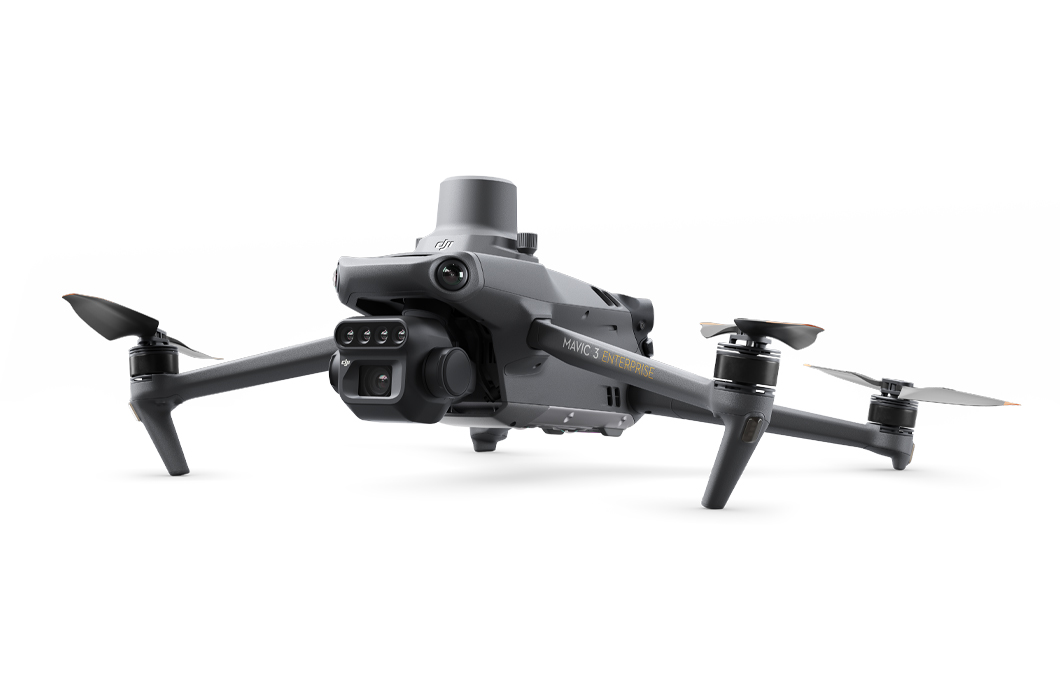
Drone based systems offer a cost-effective and customizable multispectral imaging solution. They can be operated by a single person or programmed for autonomous surveying. Given their affordability, flexibility, and ease of operation, drone-based multispectral imaging has become the preferred choice for small to medium-sized land surveying applications over the last years.
Ground Vehicle
Both satellite and aircraft-based imaging systems offer excellent imaging capabilities with good resolution. However, there are scenarios where their use is impractical or insufficient, such as indoor environments, areas under tree coverage, or situations requiring more localized insights. In these situations, ground vehicles are used such as rovers. Rover platforms provide exceptional spatial resolution, allowing for detailed analysis of crop health, stress levels, and nutrient status. Their close-up view enables early detection of issues such as disease outbreaks or nutrient deficiencies, enabling farmers with timely intervention opportunities.
In addition to their imaging capabilities, ground-based spectral imaging systems offer the unique advantage of being able to actuate based on the information collected. This means that rovers can not only gather data but also perform tasks autonomously, such as removing unhealthy crops and weeds, precisely applying pesticides, or customizing fertilizers and nutrients for each crop specimen. This level of precision enables the automation of various farming tasks, including weeding, crop monitoring, fertilization, and even harvesting, leading to improved efficiency and resource utilization. Such systems are already being developed and deployed by new companies such as Naïo Technologies with their Dino robot (depicted in the image below), or Verdant Robotics with the Sharpshooter. Legacy companies such as the ONE SMART SPRAY from Bosch or the See & Spray Ultimate from John Deer.
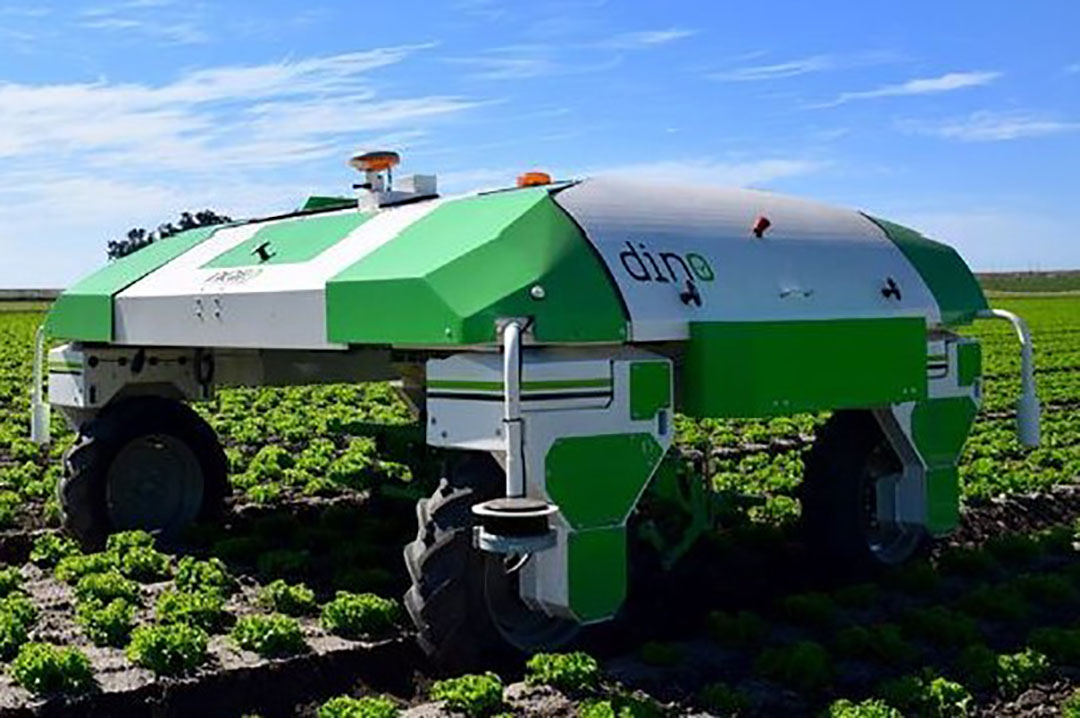